
There are benefits to both, but neither in a panacaea. I rarely see them as competitors on the same problem, and have used them on (different) real problems - often there will be a natural choice of which to look at. Permutation tests can work on small samples (though limited choice of significance levels can sometimes be a problem with very small samples), while the bootstrap is a large-sample technique (if you use it with small samples, in many cases the results may not be very useful). exact coverage of intervals) from the outset, and instead focuses on trying to get reasonably good coverage in large samples (sometimes with less success than may be understood if you haven't checked, don't assume your bootstrap gives the coverage you expect it to). In some of the more complex cases, bootstrapping is easier to do because (looking at it from the testing point of view) it operates as well under the alternative as the null (at least naive implementations will be - doing it so that it works well may be much more complicated).Įxact permutation tests can be difficult in the more complex cases because a suitable exchangable quantity may be unobservable - often a nearly-exchangeable quantity may be substituted at the price of exactness (and of being truly distribution-free).īootstrapping essentially gives up on the corresponding exactness criterion (e.g. In many cases - especially simpler ones - they're almost exactly equally easy - it's essentially the difference between sampling with replacement and sampling without replacement. In some other application areas, permutation tests would rarely be used, but the varying popularity across application areas sometimes says more about the local culture of whichever area than usefulness. These are all permutation tests (on the other hand there are many instances where permutation tests of the original data could be used instead but are usually not). How would we judge popularity? If we look at fields like psychology and education we can find plenty of use of rank based tests like Wilcoxon-Mann-Whitney, the signed rank test, rank-correlation tests and so on. (Each can be adapted to be used for the other task though.) My question is which resampling technique has gained the more popularityīootstrapping is mostly about generating large sample standard errors or confidence intervals permutation tests as the name suggests are mostly about testing. You can do a test of hypothesis this way but it tends to be less powerful than the permutation test for cases that the permutation test assumptions hold. The bootstrap estimates the variability of the sampling process and works well for estimating confidence intervals. The Mann-Whitney/Wilcoxon test is actually a special case of a permutation test, so they are much more popular than some realize.
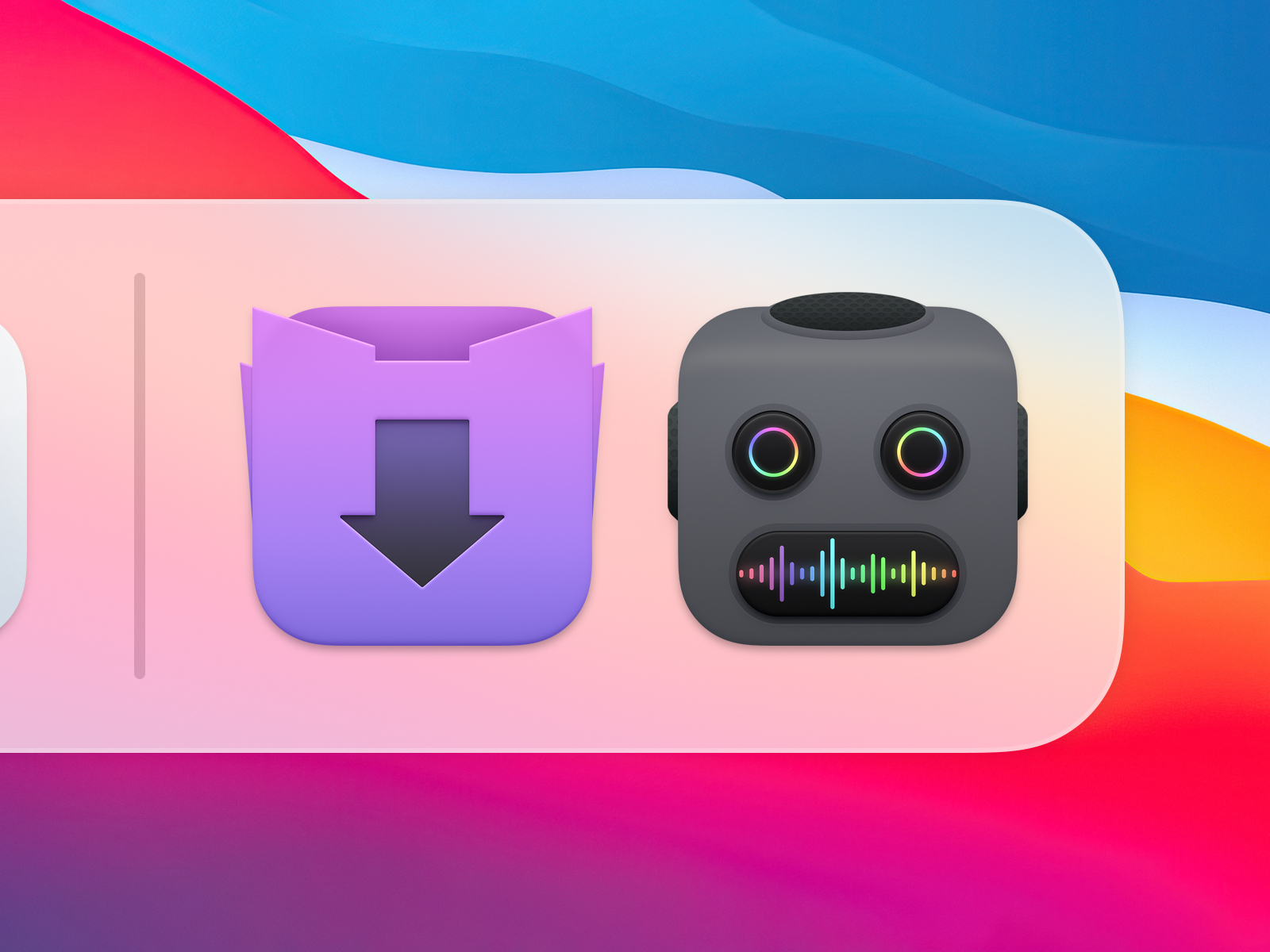
Permutation tests can be used to create confidence intervals, but it requires many more assumptions, that may or may not be reasonable (so other methods are preferred).
#Permute app srvices add url serial
It can also be expanded to things like time series (null hypothesis that there is no serial correlation) or regression (null hypothesis of no relationship). This is the common case for things like t-tests and ANOVA.
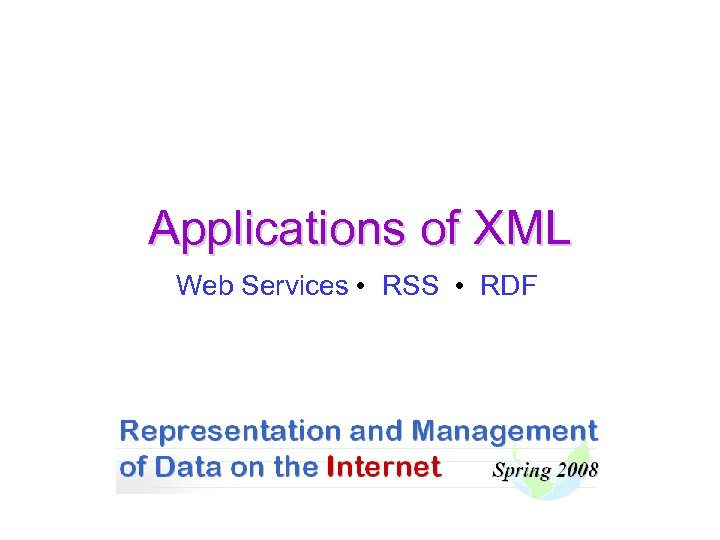
that only the random sampling/randomization explains the difference seen.

Permutation tests test a specific null hypothesis of exchangeability, i.e.

The permutation test is best for testing hypotheses and bootstrapping is best for estimating confidence intervals. Both are popular and useful, but primarily for different uses.
